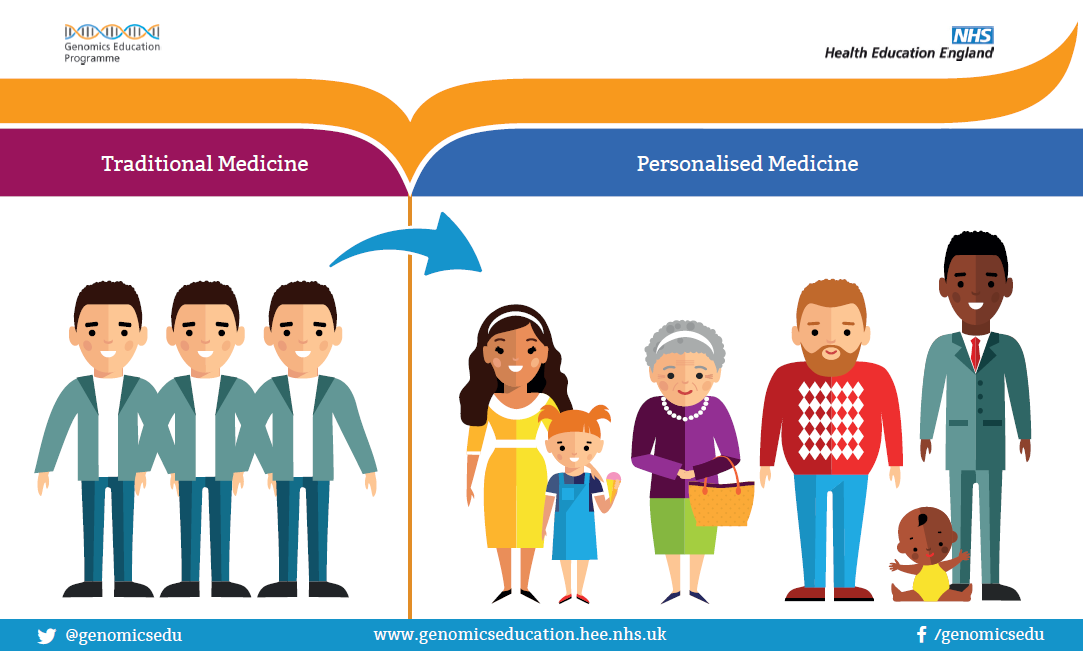
In the final of our posts regarding the recent major international evaluation of AI in breast cancer screening, we consider how AI could be used to personalise breast cancer screening in the UK.
Much focus has been placed in recent years on using polygenic risk scores to personalise cancer screening, but – to us – the potential of AI to predict medium-term risk of breast from mammograms looks to be even greater.
Cancer now versus cancer soon
The paper provided results (in terms of ROC curves) both for whether a woman would develop breast cancer in 3 years (39 months) and in 12 months. The authors’ reason for including cancers detected on the next screen was to allow the AI system to detect cancers missed by screening (on the initial mammogram). Nevertheless, one reason for the poor sensitivity of both humans and the AI system could be that many of the cancer found within 39 months of screening just weren’t present (or weren’t visible) on the original images.
What is striking is the ability of the AI system to predict cancers that were not detected within 12 months of the initial screen. What we are not told is whether those cancers were visible on the original mammograms or whether the AI system has identified a high-risk phenotype that suggests that breast cancer, whilst not detectable now, will develop over the next 3 years. One obvious candidate for such a phenotype is breast density, but detailed data provided in the paper suggests that whatever it is that the AI system is detecting is rather more complex than breast density.
Oddly, the AI system also seems to be able to detect cancer diagnosed within 12 months, without being able to correctly localise the tumours (i.e. without correctly identifying where the cancer is within the breast). At a specificity of 90% the AI system had a sensitivity of about 70% in images from women in the US, but correctly localised about 55% of cancers. Thus, of the screen-detected cancers identified by AI, nearly 20% were not correctly localised.
It is critically important to understand how the AI system is able to correctly identify women with cancer even without correctly identifying where the cancer is. And it’s even more important to understand how it is able to predict who will develop cancer over the next three years if indeed there are some women whose cancers at three years were predicted by the AI system but, even with the benefit of hindsight, were not visible at baseline.
A 5-level classification of the AI system
Based on the ROC curves in the paper, we have divided the AI system score into a 5-level risk of breast cancer (Table). For each level we give the proportion of screened women in that level and the risk of breast cancer diagnosed over the next 39 months (including on the next screen).
Level | Description | Proportion of screened women | Prevalence of breast cancer (over 39 months) |
1 | Extremely low risk | 40% | 1 in 750 |
2 | Very low risk | 40% | 1 in 150 |
3 | Average risk | 13% | 1 in 63 |
4 | High risk | 7.3% | 1 in 14 |
5 | Very high risk | 0.6% | 7 in 8 |
Overall (in the study) the risk of breast cancer within 39 months was 1 in 63 and the risk of screen-detected breast cancer (i.e. within 12 months) was 1 in 96.
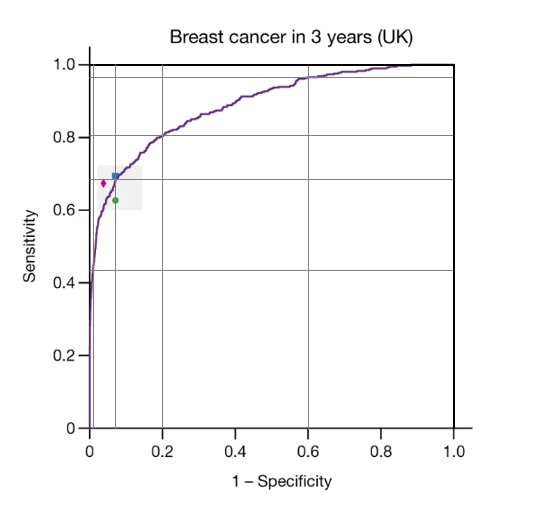
Based on the risks at each level, we recommend:
- Women at extremely high-risk (level 5) are referred for diagnostic work-up
- Women at high-risk (level 4) are assessed by a radiologist and offered further investigations
- Women at average risk (level 3) are re-invited for screening at a shorter interval: 18 months (or maybe 12 or 24 months). Note that risk in these women is the same as in women who have not been screened.
- Women at very low risk (level 2) are re-invited at the usual screening interval (3 years)
- Women at extremely low risk (level 1) are re-invited at an extended screening interval: 4.5 years (or maybe even 6 years).
It should be noted that 83% of the cancers found in women in levels 4 and 5 were found initially. We conjecture that many of those found as interval cancers or on the next screen, were in fact screen-detectable initially but were missed. It would be interesting to re-evaluate their mammograms to see if, in hindsight, there was evidence of a tumour co-located with the cancer eventually diagnosed. By contrast, the minority (44%) of those in level 3 and just 15% of those in level 2 were diagnosed within 12 months. The hope is that by re-screening level 3 women sooner, the missed cancers will be detected earlier before they have advanced to have worse prognosis or require chemotherapy.
Just 15% of those in level 2 and none (based on small numbers) in level 1 were diagnosed within 12 months. Indeed, it is possible that all cancers in level 1 women were found on the next screen at 36 months. It would be interesting to know whether they were all early stage. Greater confidence regarding the safety of an extended screening interval in level 1 women would come from knowing the size and nodal status of those cancers that were diagnosed in these women. Extended follow-up data (to include a third screen at about 72 months) would provide added confidence regarding the safety of extended screening for those in level 1.
What next?
This paper did not report on sensitivity separately by clinical stage but, particularly for cancers detected on the next screening round, it is critical to know their stage. It could be argued that if a tumour was missed initially but found as a small node-negative cancer on the next screen no harm has been done (treatment will be the same and prognosis is still excellent), whereas if the missed cancer was eventually diagnosed as stage III there would be great concern.
This paper was based on a retrospective analysis of mammograms from breast screening in the UK and the USA. The next step must surely to be to implement AI analysis of mammograms in routine screening. Initially, one should be cautious as to how AI is used. Images from women in Levels 4 and 5 who have not been referred for further testing should be re-analysed (by an expert radiologist). Mammograms from women in Level 1 that were classified as normal by a human reader need not be further reviewed. But the proposed management should also be noted: If the rate of cancer detection over the next 39 months in women in Level 1 is low enough, their screening interval can indeed be lengthened; If the cancer pick-up rate from use of a second reader in women in Level 2 is indeed very low then a second reader is not needed; And if the cancer pick-up from the first reader in Level 1 women is extremely low, then the human reader is not needed for these images.
Many people will be suspicious of the use of AI in breast screening, but if it is introduced first as a safety it should be possible to improve screening without risking unintended consequences.
In a future blog we will consider what breast screening might look like in 2030.
The views expressed are those of the author. Posting of the blog does not signify that the Cancer Prevention Group endorse those views or opinions.
Leave a Reply