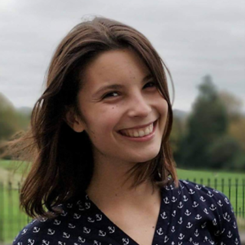
Lucie Gourmet is a third year PhD student at University College London (UCL) in the Centre for Computational Medicine. She is funded by Cancer Research UK and is part of the Alliance for Cancer Early Detection. Before her PhD she did a Scientific Baccalaureate in France before doing a MSci in Human Genetics at UCL.
Your PhD research looks at the cells and surrounding environment of cancerous tumours – the ‘tumour microenvironment’. Could you explain what you’re working on in a bit more detail?
My research has changed direction since the beginning of my PhD. In the first year, I did rotations at UCL, Cambridge and Stanford on different topics. At UCL, I was using High Resolution Episcopic Microscopy to study the tumour microenvironment. Then at Cambridge, I worked on mathematical modelling of early oesophageal tumours. Finally, at Stanford I examined the role of mutualism (cancer cells helping each other) in an agent-based model of immune evasion. After all this training and learning, I decided to create my own project.
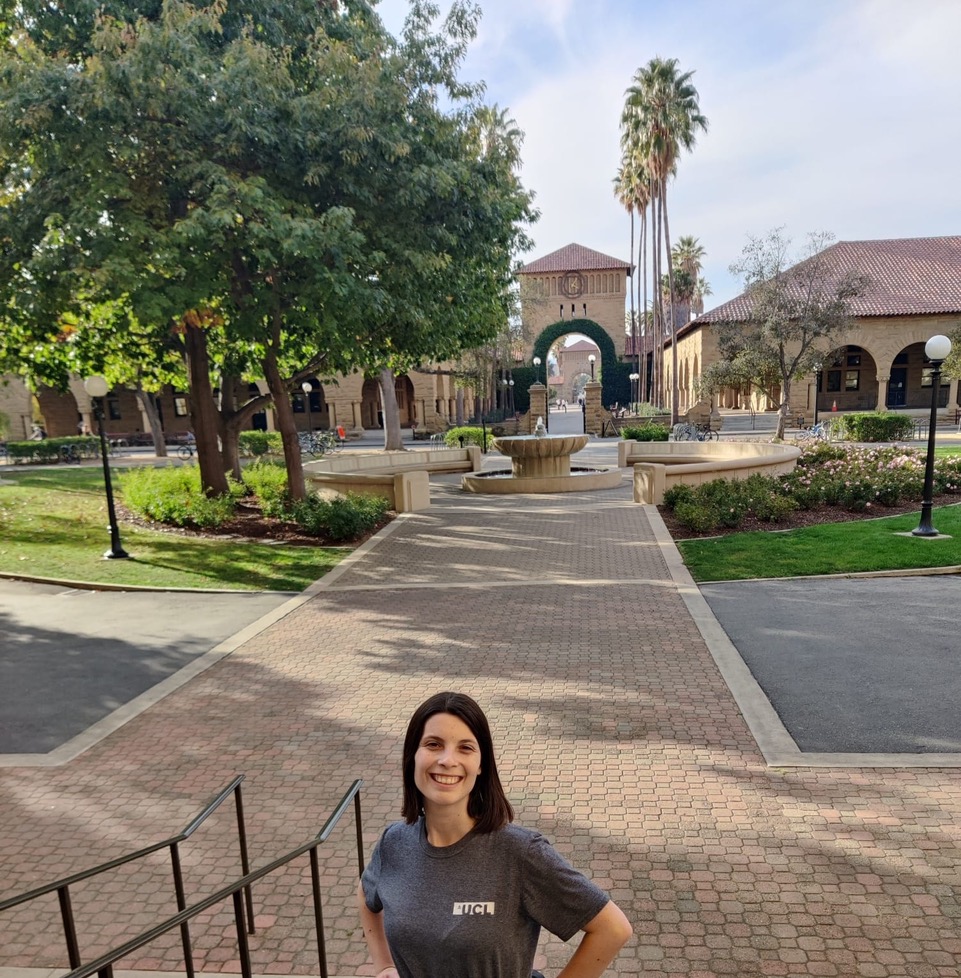
Now, I investigate how the order of mutations impacts cancer prognosis. There are 10 cancer properties which distinguish normal cells from cancer cells. These properties are called cancer hallmarks. These cancer hallmarks are found across all cancer types. They include:
- Self-sufficiency in growth signals
- Insensitivity to growth-inhibitory signals
- Evasion of programmed cell death
- Limitless replicative potential
- Sustained angiogenesis
- Metastasis
- Genome instability
- Tumour-promoting inflammation
- Avoiding immune destruction
- Re-regulating cellular energetics
My goal is to find the order in which the mutations associated with each hallmark occur.
How did you become interested in this area of research?
I was progressively drawn to cancer evolution research, through various experiences. I undertook an internship at the Mayo Clinic in Jacksonville where I had to present a paper on cancer evolutionary genetics. This made me realise that I wanted to work to answer similar questions. I also did an internship on selection pressures in cancer at the Institute of Cancer Research, which convinced me further. When I started my MSci at UCL, I chose to dive into Computational Cancer Biology. I guess I became passionate about this topic because I agree with the quote “nothing in biology makes sense except in the light of evolution.”
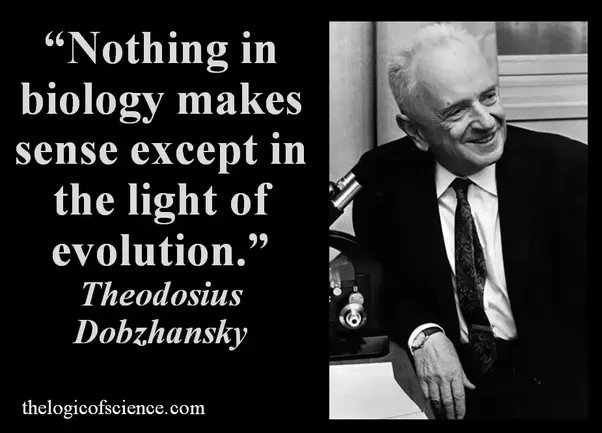
More specifically, I realised that I wanted to focus on fundamental cancer science. The hallmarks of cancer have been extensively characterised from different points of views (genomics, epigenomics, transcriptomics, proteomics). However, I could not find a paper explaining which hallmarks occurred first, so I decided to answer this question myself.
What methods do you use for this? Has your field of research changed as new diagnostic tools and technologies have developed?
Glossary of terms relevant to the following paragraph:
- Allele: one of two or more versions of DNA sequence at a given genomic location
- Mutant alleles: Alleles (versions of DNA) that no longer produce the same level or type of active product as the wild-type allele (the allele that is present most commonly in wild and natural populations)
- Somatic cells: cells that make up all the internal organs, skin, bones, blood and connective tissue
- Somatic mutations: any alteration at the cellular level in somatic tissues occurring after fertilization
We have used Variant Allele Frequency (VAF), which is the number of mutant alleles divided by the total number of alleles. The mean VAF of somatic mutations in various cancer hallmark-associated genes is estimated as a proxy for timing. In other words, hallmarks selected early will exhibit higher allele frequencies.
We have also looked at dN/dS, which is the ratio of non-synonymous to synonymous mutations, to measure selective pressures. If there is an excess of non-synonymous mutations (which alter the amino acid sequence of a protein), the dN/dS value will be greater than 1 and the gene will be considered to be under positive selection. However, if there is a depletion of non-synonymous mutations, the dN/dS value will be less than 1 and the gene will be considered to be under negative selection.
VAF and dN/dS are both important metrics in my field of research, as they provide key information on different aspects of cancer evolution. In our study, we created a simple metric combining them in order to create a more holistic approach to cancer genetics. Nevertheless, more research should be done to encompass both mutational timing and selection pressures.
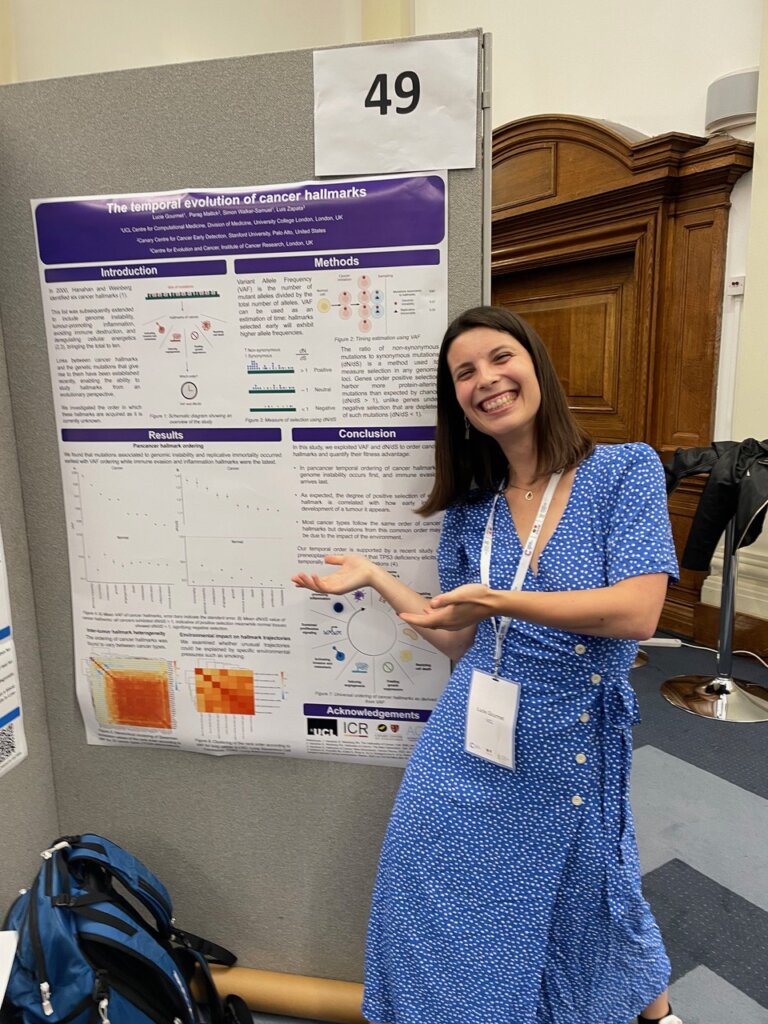
What has your research found so far?
We uncovered the order of hallmarks per patients, per cancer type and also for ‘pan-cancer’ analysis (working across 32 cancer types). When looking at pan-cancer, we found that mutations associated with genome instability are the first to emerge. This makes sense as we know that cancer is caused by the accumulation of mutations in cells. In contrast, inflammation and immune evasion were the last to occur.
Interestingly, most cancer types followed a common trajectory, whereas only five showed deviations (including skin and uveal (eye) melanoma). As skin and uveal melanomas are known to be influenced by UV light exposure, we hypothesized that specific environmental factors could affect the evolutionary trajectories of cancers. Therefore, we looked at how the hallmark order varied in lung cancers depending on smoking status (smokers, reformed smokers, non-smokers). Then, we clustered patients into 2 groups based on whether the genome instability hallmark was ranked as ‘early’ or ‘late’. We discovered that the group of patients with early genome instability had significantly worse survival. When we removed TP53 (the most commonly gene mutated in cancer) from the pan-cancer dataset, we found that genome instability became the last hallmark to emerge. By contrast, most other hallmarks were unaffected. This result suggests that early acquisition of genome instability is mainly driven by TP53.
How about adjacent work linked to this? What other work is going on in the field?
While we have been studying the temporal evolution of cancer hallmarks, but another group recently analysed the spatial distribution of cancer hallmarks. Using spatial transcriptomics, they showed that the activity of cancer hallmarks is variable across cancer cells and the tumour microenvironment. I think that the main challenge for our field is to merge biological data with medical imaging.
Are there any new areas, or questions, that have emerged from this work? What do you think the field might be working on in 10-20 years’ time?
Our work is important for personalised medicine: if we understand the tumour’s past, we can predict its future. Moreover, our research could inform therapy and help to detect cancer earlier: if we know which are the first genes to trigger cancer, we can target them.
We now know that environmental factors can alter the order of cancer hallmarks and lead to worse prognosis. Thus, we can improve survival through cancer prevention strategies related to sun exposure, smoking or diet. Hopefully, this will lead to practical changes. In fact, I am currently doing an internship with a cancer early detection start-up to see how we can implement diagnostic and prognostic tools. I believe that we require an approach combining both multi-omics (from genomics to metabolism) and imaging in order to detect, understand, and treat cancer.
The views expressed are those of the author. Posting of the blog does not signify that the Cancer Prevention Group endorses those views or opinions.